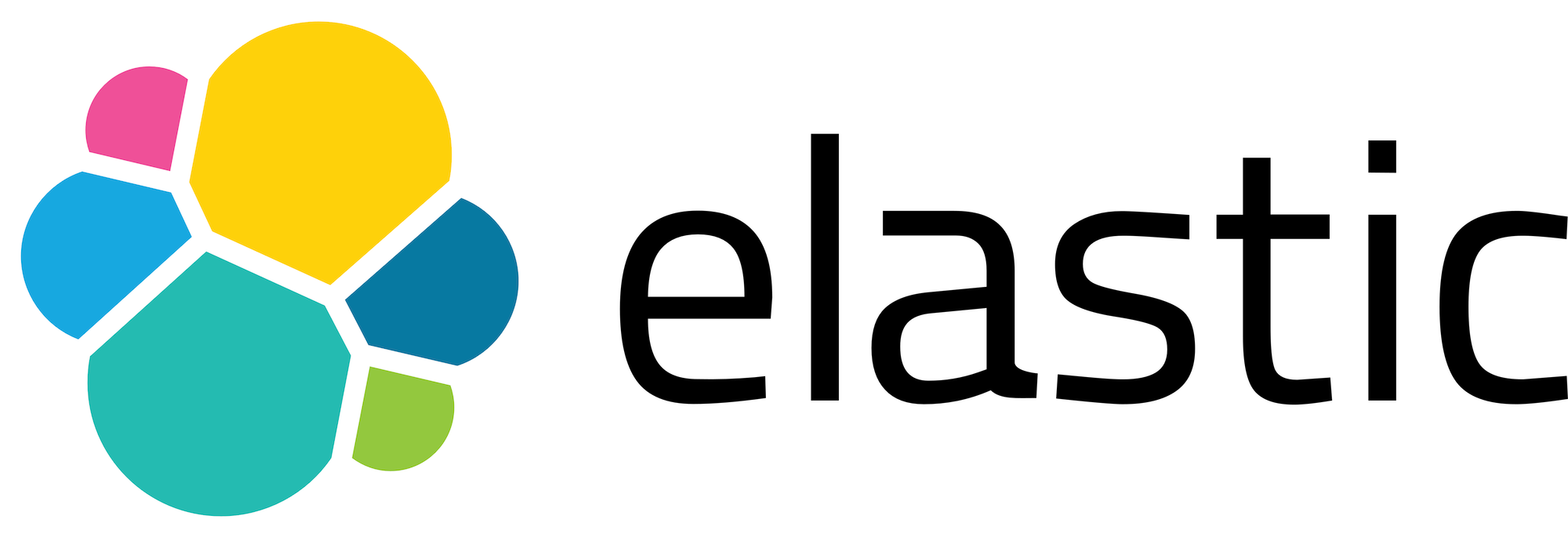

Artificial Intelligence for IT Operations (or AIOps for short) continues to be a hot topic among developers, SREs, or DevOps professionals. The case for AIOps is especially crucial given the expansive nature of today's observability efforts across hybrid and multi-cloud environments. As with most observability challenges, AIOps starts with telemetry data: metrics, logs, traces, and events.
Once IT operations teams collect and begin to analyze the data, the benefit of AIOps becomes rapidly clear. AIOps aims to accurately and proactively identify areas that need attention and assist IT teams in solving issues faster. As human beings, we cannot keep up with analyzing petabytes of raw observability data. Adding AIOps delivers a layer of intelligence via analytics and automation to help reduce overhead for a team. Let's dive in to answer common questions on this critical topic.
What Is AIOps and How Can It Help Me?
Simply put, AIOps is the ability of software systems to ease and assist IT operations via the use of AI/ML and related analytical technologies. AIOps capabilities can be applied to various operational data, including ingestion and processing of log data, traces, metrics, and much more.
Seeking to clarify the often murky and confusing world of AIOps are Gartner, Forrester and others who provide market definition. AIOps can help significantly reduce the time and effort to detect, understand, investigate, determine root cause, and remediate issues and incidents faster. Saving time during troubleshooting can, in turn, help IT personnel focus more of their energy on higher-value tasks and projects.
Why Do You Need AIOps as Part of Your Observability Strategy?
Many recent articles (Gartner glossary for AIOps, Forrester AIOps reports) describe the dynamics in the IT market. From digital transformation initiatives to cloud migration to distributed, hybrid, or cloud-native application deployments, these dynamics are dramatically changing the IT operations landscape.
The landscape changes have the following three characteristics:
■ Data volume: The volume of data for observability continues to increase exponentially.
■ Complexity: Applications, workloads, and deployments continue to become more complex, ephemeral, and distributed.
■ Pace of change: The rate at which changes (application and infrastructure) occur is faster than ever before.
These are not mutually exclusive. In some ways, quite the opposite. For example, high rates of change and complex deployments utilizing auto-scaling mean an even higher volume of data. This increasing complexity means that humans will depend on systems and automation to keep up with the changes. For this reason, AIOps will play a key role in responding to these operational and business challenges.
Leveraging AI/ML to roll up data, summarize it, and intelligently tier the data for storage can help alleviate some of the volume challenges. Explicit visual depictions of an application environment (infrastructure and service dependency maps) and contextual navigation help align troubleshooting efforts with how users think of their deployment. Furthermore, auto-surfacing of problems and root causes analyses will address some of the other complex challenges.
Observability products will need to keep track of all application and infrastructure changes and correlate those changes with system behavior and user experience because change is often the root cause of acute, anomalous behavior. An upgrade or patch for a new feature with unintended consequences is a typical example. Enabling those correlations helps teams be more agile and adept at keeping pace with those frequent changes helping sustain service performance.
AIOps play a key role and can help navigate these challenges effectively, freeing up operations teams to focus on more important work when properly implemented and used.
Which Observability Use Cases Are Best for AIOps?
Several observability workflows and use cases are already very well served with the application of AIOps techniques and technologies, for example:
■ Service degradation such as sudden or unexpected variations in latency can be detected via anomaly detection.
■ Massive volumes of data, such as unstructured or semi-structured log messages, can be automatically classified, categorized, and summarized to help ease consumption and analysis.
■ Multiple symptoms, events, and issues can be correlated to help cut down alert "noise" and reduce time to root cause determination.
■ Automatic health scoring based on an assessment of impact, the extent of anomalies, and other measures help surface the most critical issues first, further reducing noise.
In the more well-understood and time-tested "if this is the symptom, then this is the likely root cause" relationships, AIOps can help automatically look for, detect, and classify those symptoms and surface those potential root causes. Ultimately, AIOps can enable remediation actions to fix routine or trivial issues and reduce burnout for operations teams
In a future blog, we will dive deeper into key use cases and how you can identify scenarios to apply AIOps in day-to-day operations.