
Datadog announced multiple product launches, including expanded monitoring capabilities for BigQuery.
Datadog’s expanded BigQuery monitoring capabilities, now in preview, help teams view BigQuery usage by user and project to identify those incurring the most spend, pinpoint the long-running queries in those segments to optimize, and detect data quality issues.
“BigQuery is an integral part of Google Cloud users’ tech stacks, enabling them to unlock insights from their proprietary datasets. With Datadog’s new monitoring capabilities, Google Cloud customers can more granularly track usage, attribute costs to users and teams, and ensure their BigQuery data is up to date for accurate insights,” said Yasmeen Ahmad, Managing Director of Strategy & Outbound Product Management for Data, Analytics & AI at Google Cloud.
“Today, it takes significant time to pinpoint where the largest BigQuery usage is coming from across projects and drill into the problematic queries to optimize. With our new BigQuery monitoring capabilities, which complement our existing 35+ Google Cloud integrations, Datadog customers can identify cross-project BigQuery cost centers, high-impact optimization opportunities and the stakeholders that need to be involved,” said Yrieix Garnier, VP of Product at Datadog. “Customers can also improve data quality by detecting data freshness and volume anomalies so they can fix issues quickly and ensure their business has accurate and up-to-date insights.”
Datadog’s expanded BigQuery monitoring capabilities build on the company’s existing capabilities for Google Cloud. Other recent product launches and integrations with Google Cloud include:
- LLM Observability: With Datadog LLM Observability, users can monitor, troubleshoot, improve and secure their Gemini and Vertex AI LLM applications, and get started quickly with auto-instrumentation.
- Cloud TPU Integration: With Datadog’s new Cloud TPU integration, teams can detect resource bottlenecks in—and underutilization of—their TPU infrastructure across workers and GKE clusters.
- Private Service Connect: Datadog users can now send their observability telemetry to Datadog’s Google Cloud-hosted sites with Google’s Private Service Connect for better data security and reduced data transfer costs.
- GKE Autoscaling (in Preview): Datadog Kubernetes Autoscaling gives users multi-dimensional workload scaling recommendations for their GKE environment and the ability to automate them within the Datadog platform, enabling teams to deliver cost savings while maintaining performance and stability.
- Storage Monitoring (in Preview): With Storage Monitoring for Google Cloud Storage, users get visibility into their Google Cloud Storage at the object and prefix levels, enabling teams to identify bottlenecks, track performance and quickly detect unusual growth in their storage consumption.
- Google Cloud Cost Recommendations (in Preview): Datadog Cloud Cost Management now automatically identifies cost inefficiencies in Google Cloud environments and provides optimization recommendations for Google Cloud services like Cloud Run and Cloud SQL.
These capabilities further enhance Datadog’s ability to provide world-class observability and security at scale for joint customers.
The Latest
According to Auvik's 2025 IT Trends Report, 60% of IT professionals feel at least moderately burned out on the job, with 43% stating that their workload is contributing to work stress. At the same time, many IT professionals are naming AI and machine learning as key areas they'd most like to upskill ...
Businesses that face downtime or outages risk financial and reputational damage, as well as reducing partner, shareholder, and customer trust. One of the major challenges that enterprises face is implementing a robust business continuity plan. What's the solution? The answer may lie in disaster recovery tactics such as truly immutable storage and regular disaster recovery testing ...
IT spending is expected to jump nearly 10% in 2025, and organizations are now facing pressure to manage costs without slowing down critical functions like observability. To meet the challenge, leaders are turning to smarter, more cost effective business strategies. Enter stage right: OpenTelemetry, the missing piece of the puzzle that is no longer just an option but rather a strategic advantage ...
Amidst the threat of cyberhacks and data breaches, companies install several security measures to keep their business safely afloat. These measures aim to protect businesses, employees, and crucial data. Yet, employees perceive them as burdensome. Frustrated with complex logins, slow access, and constant security checks, workers decide to completely bypass all security set-ups ...
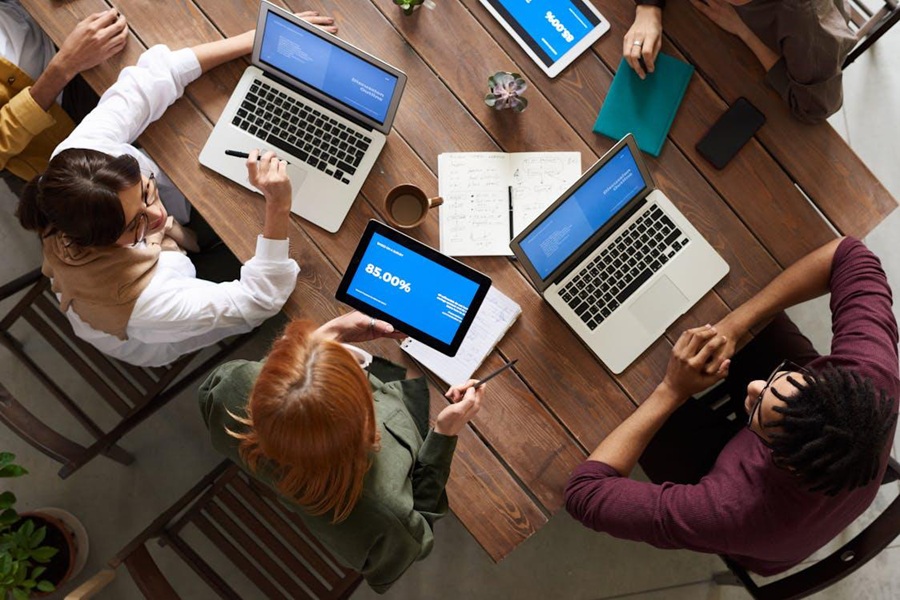
In MEAN TIME TO INSIGHT Episode 13, Shamus McGillicuddy, VP of Research, Network Infrastructure and Operations, at EMA discusses hybrid multi-cloud networking strategy ...
In high-traffic environments, the sheer volume and unpredictable nature of network incidents can quickly overwhelm even the most skilled teams, hindering their ability to react swiftly and effectively, potentially impacting service availability and overall business performance. This is where closed-loop remediation comes into the picture: an IT management concept designed to address the escalating complexity of modern networks ...
In 2025, enterprise workflows are undergoing a seismic shift. Propelled by breakthroughs in generative AI (GenAI), large language models (LLMs), and natural language processing (NLP), a new paradigm is emerging — agentic AI. This technology is not just automating tasks; it's reimagining how organizations make decisions, engage customers, and operate at scale ...
In the early days of the cloud revolution, business leaders perceived cloud services as a means of sidelining IT organizations. IT was too slow, too expensive, or incapable of supporting new technologies. With a team of developers, line of business managers could deploy new applications and services in the cloud. IT has been fighting to retake control ever since. Today, IT is back in the driver's seat, according to new research by Enterprise Management Associates (EMA) ...
In today's fast-paced and increasingly complex network environments, Network Operations Centers (NOCs) are the backbone of ensuring continuous uptime, smooth service delivery, and rapid issue resolution. However, the challenges faced by NOC teams are only growing. In a recent study, 78% state network complexity has grown significantly over the last few years while 84% regularly learn about network issues from users. It is imperative we adopt a new approach to managing today's network experiences ...
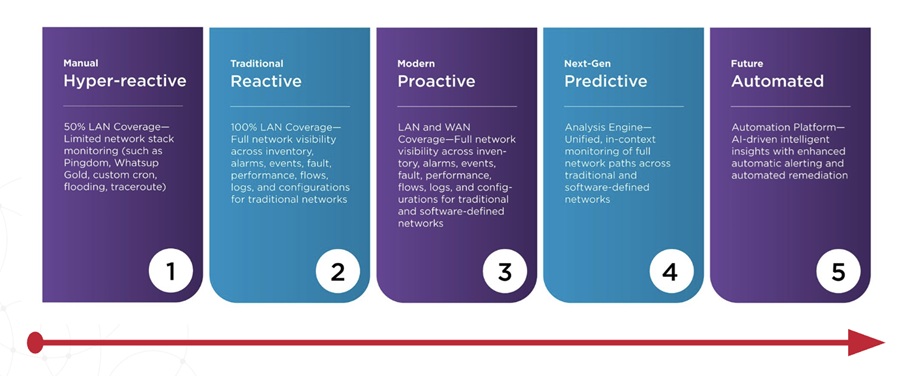
From growing reliance on FinOps teams to the increasing attention on artificial intelligence (AI), and software licensing, the Flexera 2025 State of the Cloud Report digs into how organizations are improving cloud spend efficiency, while tackling the complexities of emerging technologies ...