
Datadog announced an expanded strategic partnership with Google Cloud, which enables Google Cloud customers to proactively observe and secure their cloud-native and hybrid applications within Datadog's unified platform.
As part of the expanded partnership and integrations, Datadog integrates with Vertex AI, allowing AI ops teams and developers to monitor, analyze and optimize the performance of their machine learning models in production.
"Google Cloud continues to be a key partner for Datadog as we jointly help global businesses observe and secure their cloud applications," said Yrieix Garnier, VP of Product at Datadog. "The new Vertex AI integration expands this partnership and gives AI and ML developers full observability into their production applications built on Vertex AI. With out-of-the-box dashboards and real-time monitors, customers can get started quickly and ensure their models are performing at an optimal level while delivering predictions responsively at scale and without errors."
"Generative AI is fundamentally changing how many businesses operate, fueling a new era of cloud that can benefit virtually every area of an organization," said Kevin Icchpurani, Corporate VP, Global Partner Ecosystem & Channels at Google Cloud. "By applying Vertex AI, Datadog can help AI teams improve how they monitor and analyze the performance of machine learning models, ensuring they are functioning correctly and creating optimal value."
Datadog's integration with Vertex AI provides developers full observability on the prediction performance and resource utilization of their custom AI/ML models. The integration provides an out-of-the-box dashboard with prediction counts, latency, errors and resource (CPU/Memory/Network) utilization grouped by deployed models so teams can compare model performance side-by-side in production environments. It also helps detect data anomalies in order to maintain the reliability and robustness of machine learning applications.
Other new and expanded Google Cloud integrations that were recently announced include:
- Serverless monitoring: Datadog now offers in-depth support for Google Cloud Run—the leading serverless compute technology on Google Cloud. With native distributed tracing across all runtimes and the ability to collect custom metrics and logs, Datadog provides deep insights into customers' Cloud Run workloads as well as fully managed APIs, queues, streams and data stores.
- Google Cloud Ready - Cloud SQL: Datadog has earned the Google Cloud Ready designation for the Google Cloud SQL integration, providing visibility into the performance and health of Cloud SQL to customers. This integration monitors throughput, memory and availability metrics in customers' databases from MySQL, PostgreSQL and SQL Server.
- Google Security Command Center: Customers can now send their Google Cloud Security Command Center findings to Datadog, including vulnerabilities, threats and errors from containers and virtual machines. Using Datadog Cloud SIEM, customers can automatically generate signals and perform investigations.
- Quick setup: Datadog's new setup experience allows Google Cloud customers to get started in just seconds so they can monitor their entire Google Cloud environment, even when there are thousands of projects. New projects can also be auto-discovered to ensure complete and seamless monitoring coverage. With out-of-the-box dashboards and real-time monitors on over 30+ Google Cloud integrations, customers can begin monitoring their services in just a few clicks.
These integrations are available now.
The Latest
According to Auvik's 2025 IT Trends Report, 60% of IT professionals feel at least moderately burned out on the job, with 43% stating that their workload is contributing to work stress. At the same time, many IT professionals are naming AI and machine learning as key areas they'd most like to upskill ...
Businesses that face downtime or outages risk financial and reputational damage, as well as reducing partner, shareholder, and customer trust. One of the major challenges that enterprises face is implementing a robust business continuity plan. What's the solution? The answer may lie in disaster recovery tactics such as truly immutable storage and regular disaster recovery testing ...
IT spending is expected to jump nearly 10% in 2025, and organizations are now facing pressure to manage costs without slowing down critical functions like observability. To meet the challenge, leaders are turning to smarter, more cost effective business strategies. Enter stage right: OpenTelemetry, the missing piece of the puzzle that is no longer just an option but rather a strategic advantage ...
Amidst the threat of cyberhacks and data breaches, companies install several security measures to keep their business safely afloat. These measures aim to protect businesses, employees, and crucial data. Yet, employees perceive them as burdensome. Frustrated with complex logins, slow access, and constant security checks, workers decide to completely bypass all security set-ups ...
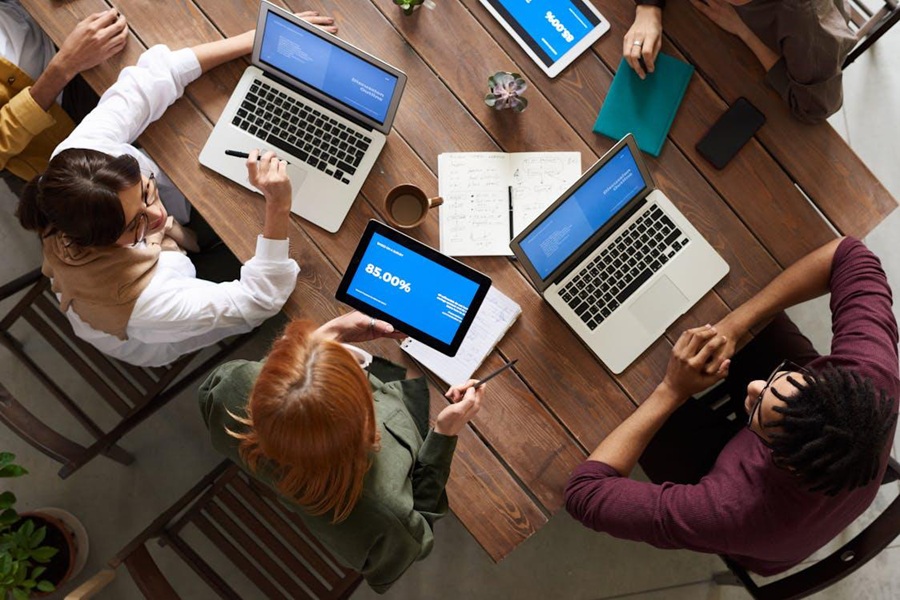
In MEAN TIME TO INSIGHT Episode 13, Shamus McGillicuddy, VP of Research, Network Infrastructure and Operations, at EMA discusses hybrid multi-cloud networking strategy ...
In high-traffic environments, the sheer volume and unpredictable nature of network incidents can quickly overwhelm even the most skilled teams, hindering their ability to react swiftly and effectively, potentially impacting service availability and overall business performance. This is where closed-loop remediation comes into the picture: an IT management concept designed to address the escalating complexity of modern networks ...
In 2025, enterprise workflows are undergoing a seismic shift. Propelled by breakthroughs in generative AI (GenAI), large language models (LLMs), and natural language processing (NLP), a new paradigm is emerging — agentic AI. This technology is not just automating tasks; it's reimagining how organizations make decisions, engage customers, and operate at scale ...
In the early days of the cloud revolution, business leaders perceived cloud services as a means of sidelining IT organizations. IT was too slow, too expensive, or incapable of supporting new technologies. With a team of developers, line of business managers could deploy new applications and services in the cloud. IT has been fighting to retake control ever since. Today, IT is back in the driver's seat, according to new research by Enterprise Management Associates (EMA) ...
In today's fast-paced and increasingly complex network environments, Network Operations Centers (NOCs) are the backbone of ensuring continuous uptime, smooth service delivery, and rapid issue resolution. However, the challenges faced by NOC teams are only growing. In a recent study, 78% state network complexity has grown significantly over the last few years while 84% regularly learn about network issues from users. It is imperative we adopt a new approach to managing today's network experiences ...
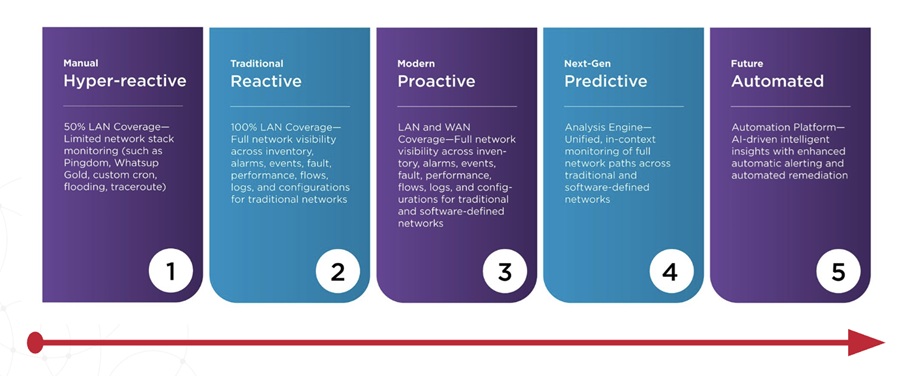
From growing reliance on FinOps teams to the increasing attention on artificial intelligence (AI), and software licensing, the Flexera 2025 State of the Cloud Report digs into how organizations are improving cloud spend efficiency, while tackling the complexities of emerging technologies ...